When I was first starting my academic lab, I worked with an executive coach who helped me find a management style that works for me and feels compatible with research science. One of my biggest confusions early on was that OKR-style management frameworks just don’t work for research science. The whole point of research is that we don’t know where we’re going or how long it will take, and so planning using deadlines and deliverables mades about zero sense!
Instead, I now think about advising research teams through the lens of ‘traction.’ The two essential ingredients for traction are:
a question or problem you’re genuinely interested to investigate
tools that are giving you new information about the universe
Having traction is the “things are moving” feeling. When you have traction on a project, every new experiment brings to mind a handful of next courses of action, many of which you’re dying to execute because you want to see what happens! In contrast, if you don’t have traction on a project, there’s no amount of work you can do that feels like it moves you closer to something worthwhile. The opposite of traction is frustration.
Having traction means different things for different people. Traction requires achieving a balance between where you want to go and how fast you’re getting there. Ambitious people often pick problems that are too big to get traction with the tools that are available (I’m prone to this failure mode). You always need to budget way more time than you think for learning how to use new tools, or getting them working for a new problem. But as I write about in Erika Update #12 (linked below), once you have traction it is such a delight to be able to ask and answer questions, and move forward!
When I think about giving advice to other scientists, I mostly think about it in this framework. If you don’t have traction, how do you get it? If you do have traction, where can you go next?
Erika Update #12
Read the update: Erika Update #12 - 2018 8 28 - kinetic luminescence of first batch of UAGA and XYZZ
Now that I had a reliable method for quantifying the activity of quadruplet-decoding tRNAs, I was off to the races! I was able to apply the measurement technique I’d developed to all the qtRNAs I’d developed to date, and classify them as Extremely Toxic, Nonfunctional, Minimally Functional or Functional. As with any new experiment when you have traction, this data generated more questions:
I had done a good job selecting qtRNAs to test and had starting points for evolution for 11/20 canonical amino acids. What about the other 9? I spent a ton of time puzzling over this data, reading the literature, and scheming about what to test next.
Time for evolution! In fact, there were now more qtRNAs to evolve than I could fit in a standard evolution experiment.
Where’d it end up?
This is where it gets exciting. This data got published! The way I was collecting this data was reliable enough that every little bit of data I collected counted. When I published papers years later I had a whole data management scheme that allowed me to include all measurements I had ever taken - across many different days and years - into my final calculations. In this update, I simply classify each sample as Extremely Toxic/ Nonfunctional/ Minimally Functional/ Functional, but the full data (as shown on the first page) was all saved and appears in the appropriate parts of all. these. figures. in. two. different. papers. !!
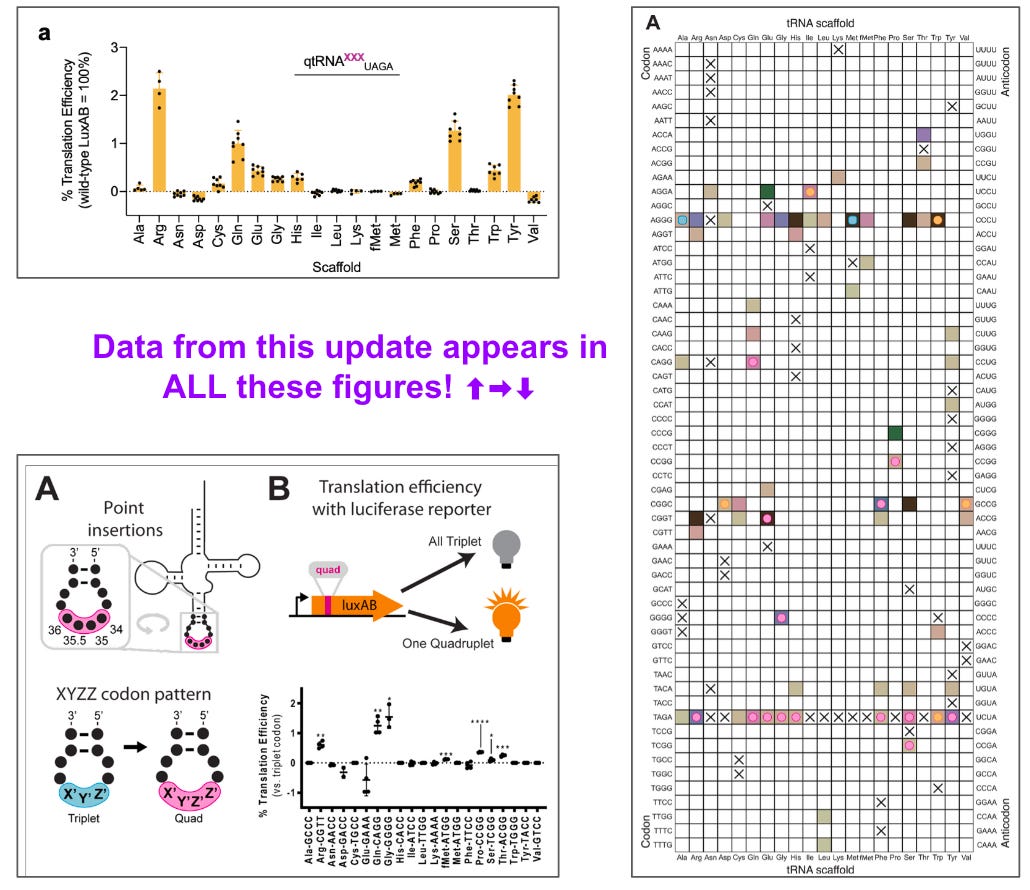
Want more?
If you want to follow along with this project, you can get updates by signing up through substack, or following me on linkedin or twitter.
If you have ideas for what I should cover in the blog post, suggestions for vocabulary to define, questions about the science, or other comments, please do reach out by twitter DM - I’d love to hear from you!