Erika Update #2: Three-out-of-four is pretty good reproducibility in life science
View the literature with skepticism
Standing on the shoulders
When I began my main project in graduate school, I was very fortunate to stumble upon an excellent 2001 paper by Thomas Magliery that served as a jumping off point for my research. This paper is awesome in so many ways: the experiments he did are explained clearly, his logic is systematically laid out and easy to follow, and he has good diagrams and data. Most importantly, he tried all the things you wish someone had tried! Finding a paper like this is such a gift to someone following up on the original work. It offered a clear place to start.
I wanted to pick up where this guy left off. I decided to try to replicate some key results from his Table 1 by testing four of the tRNAs he reported decode four-base codons. As I describe in Erika Update #2 (linked below), only three out of four of the tRNAs work in my hands. One of them, the CUCU one (the two pink bars, and then the two red bars on the last page of the Update) doesn’t work for me even though it worked for Magliery.
I often tell people this anecdote as an example of reproducibility in life science. 3/4 is pretty good reproducibility in biology! It says a lot about the complexity of the system we’re dealing with. Why does the CUCU qtRNA work differently for me than it worked for a guy I’ve never met 20 years ago? My best guess is that he was using a different strain of E. coli that has subtly different properties, and this causes it to treat the CUCU transcript and qtRNA different somewhere: when the tRNA is chemically modified, when the AARSs do proofreading, when it interacts with the mRNA transcript in the ribosome, when the ribosome checks codon anticodon matching - something somewhere was different in his strain of E coli than in mine today. But it’s basically impossible to figure out what’s different when I don’t have access to his samples, which I’m sure are long gone.
There’s a lot more to be said about reproducibility in life science. Irreproducibility has many sources. Sometimes, it is a clue that there’s more to learn on a basic science level.
Erika Update #2
As a quick recap, this post is part of the “Erika Updates” series, where I post informal research updates I wrote in grad school accompanied by blog posts with commentary. I’m revisiting these write ups as a jumping off point for reflecting on the PhD experience, the current state of research science, and the ways we share and communicate technical content to peers and the public.
Last time I made a reporter, and this time I used the reporter to test out more tRNAs, a type of biomolecule I wanted to engineer, and measure how good they are at decoding quadruplet codons.
Here it is 2018 3 6 - S AGGA UAGA anticodon loop side reversion
On the first page of the Update, I talk about how Magliery had identified some mutations to serine tRNAs that make them better at decoding quadruplet codons, which was exactly what I wanted to do! Those results replicate. Having examples of a ‘worse’ and a ‘better’ tRNA ended up being incredibly useful for validating my engineering process for going from one to the other.
On the second page, there’s also some foreshadowing about the upcoming struggle with toxicity. As I try moving away from Magliery’s results to experiment with tRNAs with different anticodons, one of the first things I noticed is that expressing some of these qtRNAs slows down bacterial growth a lot. That problem becomes more and more apparent as the project progresses…
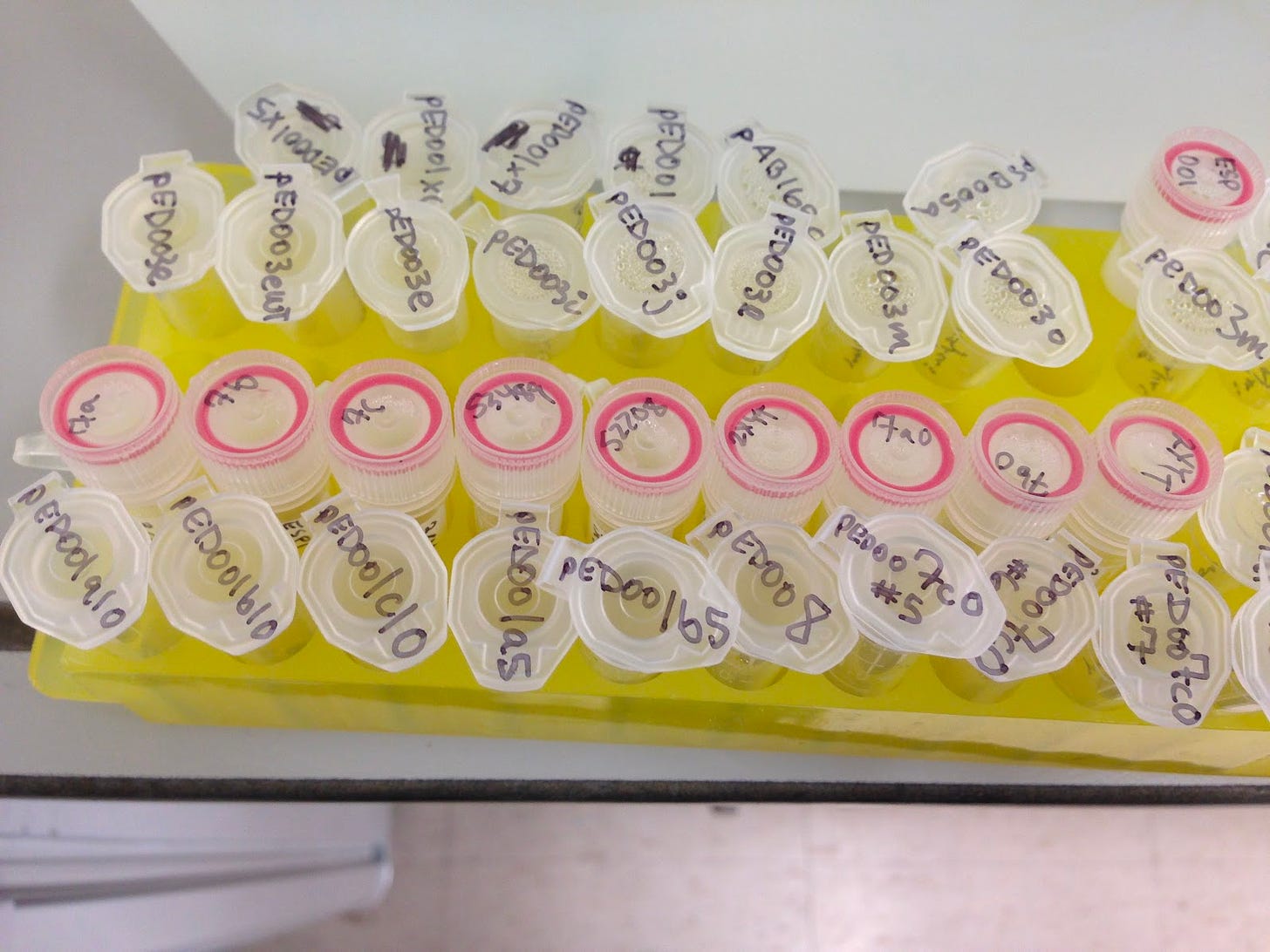
Where’d it end up?
Like the last update, this data was very central to the papers after it was re-collected using an improved technique I hadn’t yet developed. The data that relates codon occupancy and some growth metric (in this case, ability to clone) is really interesting, but never ended up anywhere. It’s not a very refined or quantitative way to characterize the relationship.
And to orient you
I think pretty much everyone can get something out of reading these blog posts + updates. Here’s some additional notes customized for you, depending on your interests + background:
You’re a non-scientist: In this project, I’m trying to engineer a type of biomolecule called a tRNA. In the previous Update, I established that I can measure (“yes” or “no”) whether different tRNAs work or not. This time, I show that I can measure whether they work “not at all”, “a little”, or “a lot!” The sets of three blue and red bars on the graph on the first page are both examples of “not at all”/“a little”/“a lot” measurements.
You’re a student doing a PhD/undergrad research/etc: It is usually worthwhile to approach the literature with some skepticism. Even the best papers in life science may be using slightly different strains, reagents or equipment than you are, and it can impact the results in ways nobody expects. It’s worth double-checking thoroughly yourself before assuming other people’s results will hold.
You have ideas for reforming publishing: Now we have a science nugget that’s provocative. Why did only 3/4 of Magliery’s tRNAs work in my hands? Today, there is absolutely nowhere to make this information known to your scientific peers. You can’t publish it in a traditional setting because it’s a small negative result. But you can imagine that it would be incredibly useful for papers in the literature to be annotated by people who can report on whether or not they get similar results when they attempt to replicate findings. In this case, doing so might give us more clues about why the CUCU qtRNA worked for Thomas but not for me.
You’re a fellow PACE nerd: A great way to validate a new reporter/circuit architecture is to engineer a worse version of your biomolecule and confirm that your circuit can tell it apart from the original. This is part of the wind-up to PACE. The next step is starting with the worse genotype and confirming you can recover the original using evolution.
You’re a fellow genetic code expansion nerd: why do mutations to the sides of the anticodon loop improve translation of quadruplet codons? There’s a fascinating 1982 paper that identifies patterns in how the anticodon is related to the bases on the sides of the anticodon loop that gets at this topic.
Vocabulary highlight: “tRNA/anticodon”
I had a brief explanation of this in a previous post, but here’s the concept behind tRNAs and anticodons in a bit more detail.
tRNAs are adaptor biomolecules. They carry an amino acid attached to their CCA tail at the “top.” They have an anticodon at the “bottom” in the anticodon loop. Codons and anticodons are geometrically compatible - like puzzle pieces. Each codon can attach to the complementary anticodon through Watson-Crick base pairing. The tRNAs, together with proteins called Aminoacyl tRNA Synthetases (AARSs) that help attach amino acids to tRNAs, dictate the genetic code.
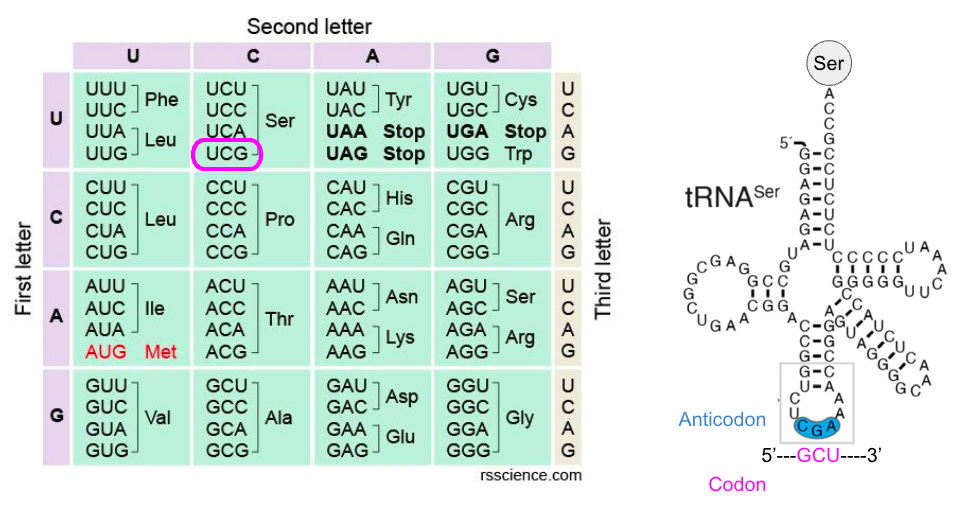
Above is the normal genetic code, and example of a normal tRNA that implements one of the codons. The serine tRNA shown here implements the “UCG” box in the codon table. The codon “UCG” and the anticodon “CGA” are reverse complements of one another.
I’d like to say that each organism has exactly 61 tRNAs, one for each non-stop codon. Unfortunately biology decided it would be more complicated! There are two reasons:
tRNAs can “wobble base pair” with codons, allowing a single tRNA to bind anticodons that sometimes differ at the third base in the codon. For this reason, just one or two tRNAs can often serve all four codons in one of the mint green boxes above with the same amino acid.
Organisms often encode more than one copy of their essential genes, so some tRNAs are present in the genome a few times.
As a result, each organism has at least 20 different tRNAs, which is at least one for every canonical amino acid.
Want more?
Please do give me feedback on these posts! Do you have requests for vocabulary highlights? What do you want to hear about in “And to orient you”? Other questions or comments? Please do reach out by twitter DM - I’d love to hear from you!
If you want to follow along with this project, you can get updates by signing up through substack, or following me on linkedin or twitter.
Hi Dr Erica,
Would like to appreciate the writing through the stories! I enjoy reading from chapter to chapter :)
In addition, I am wondering with your previous experience with tech and so forth, why did you finally choose to do bio? What brings you specifically working on something specific such as tRNA, genetic code expansion and Aars? Do you envision something related to your career aspirations to this day? (I read also about automation and reproducibility that you mentioned in your essays)
Thanks in advance!
Aditya