Erika Update #7: Evolution is a tightrope walk
Success is a balance between extinction and complacency
Humanity has a long history of using evolution as an engineering tool. Breeding dogs, cultivating roses, and domesticating crops like corn are all huge success stories in which we’ve engineered the properties of biological organisms using nothing more complicated than genetic diversification and selection.
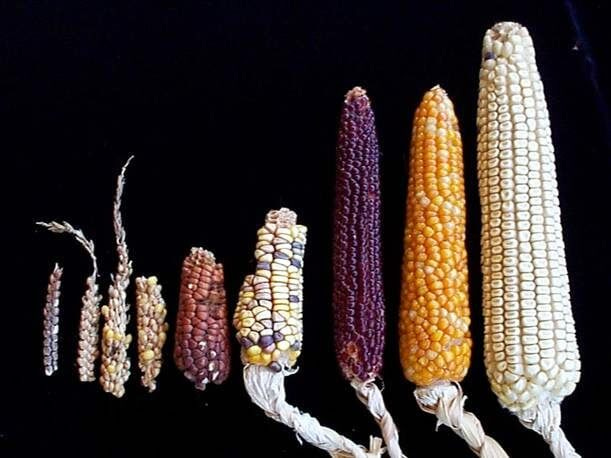
But evolution is a tricky tool. Unlike CAD software, which allows you to reliably generate a design to spec, evolution is uncertain, stochastic, and error-prone. If you’re trying to use evolution for engineering, it may just not work.
There are two main failure modes:
Extinction. If you subject a population to an environment that is too hard, it will die out before it has a chance to evolve.
Complacency. Conversely, if a population is in an easy environment, that poses little challenge, it may simply not evolve at all!
If you’re curious to hear more about these, I dive into these failure modes in a bit more detail in the talk below:
If we want to reliably use evolution to do engineering, we need to nail a ‘goldilocks’ environment every time. It needs to be hard enough, but not too hard, to force evolution to occur without risking extinction. In Erika Update #7, linked below, I’m experimenting with ways to control the stringency of qtRNA evolution. That is to say, I’m fiddling with how difficult the environment is, and identifying all the different knobs that I can turn to make it just right.
Erika Update #7
Here’s Erika Update #7 - 2018 5 3 - crosstalk - S UAGA naive vs Mag enrichment comparison
In pages 2-4 of this update, I’m experimenting with four different strategies for making the qtRNA evolution environment easier or harder. Things I have control over:
The number of quadruplet codons (more is harder)
Promoter strength (weaker promoter is harder)
Copy number of the plasmid (lower copy is harder)
RBS selection (orthogonal ribosome is harder than endogenous ribosome)
On the last page, I do a brief experiment that helps me predict whether the viruses I want to put into a long-running evolution experiment would be likely to go extinct right away, survive complacently, or be somewhere in the right ballpark. I test the viruses out in each of the environments I have at my disposal. Had I just randomly picked a long-running evolution to run there’s no way I would have gotten it right! The combinations I was experimenting with covered nearly nine orders of magnitude of difficulty, and there’s a pretty narrow window of ~2 orders of magnitude that is the ‘goldilocks’ region.
Where’d it end up?
You know the drill here… no of course it didn’t make it into a paper! This is still behind-the-scenes work. Although a couple of the plaque assay images in Figure 2c of this paper might have come from the plaque assays I did on page 4.
And to orient you
I think pretty much everyone can get something out of reading these blog posts + updates. Here’s some additional notes customized for you, depending on your interests + background:
You’re a non-scientist: Evolution in the lab gives us a little microcosm where we can try to understand how evolution on Earth works. How challenging would the environment have needed to be for the dinosaurs to not go extinct, but instead evolve to thrive on post-astroid Earth?
You’re a student doing a PhD/undergrad research/etc: On pages 2 and 3 I have lots of little diagrams with arrows indicating which of my plasmids have which properties. These diagrams don’t appear anywhere else, but they were SO useful for me to make! I made a lot of internal notes that helped me navigate my own enormous repository of constructs.
You have ideas for reforming publishing: I would actually really love to write a document that describes the workflow for how to actually get your PACE to work. There’s a lot of insider knowledge involved in knowing what strategies for tuning the environment will be successful, and what “goldilocks” looks like. There is nowhere for me to publish this.
You’re a fellow PACE nerd: At the end, we see that the “Naive qtRNASerTAGA” is just barely fit enough on the easiest AP I made. We know that increasing the copy number makes the environment less stringent, so why not increase copy number some more? We’ll come back to this in a later update, but basically you should keep copy number <50 copies/cell in order to avoid a technical issue that PACE has which is that if pIII accidentally recombine onto the phage it creates a virus that can escape selection entirely. High copy APs are more prone to generating recombinants, so keep the copy number modest.
Want more?
If you want to follow along with this project, you can get updates by signing up through substack, or following me on linkedin or twitter.
If you have ideas for what I should cover in the blog post, suggestions for vocabulary to define, questions about the science, or other comments, please do reach out by twitter DM - I’d love to hear from you!